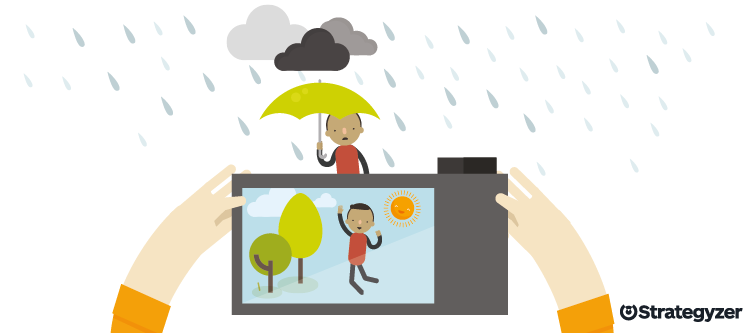
Experiments helps product managers, entrepreneurs or intrapreneurs catch a glimpse of what their market looks like. These snapshots enables them to make data-backed decisions when building a new venture. But poorly designed experiments inevitably generate an inaccurate picture of how customers actually behave.
Salim Virani, author of Decision Hacks, and Paul Mackinaw, Principal at Leancog, both helped me construct the list of 13 testing mistakes that can distort your 'market snapshots' and make your research irrelevant. Are you stuck in these traps? Check out the list and feel free to add your own traps in the comments below:
1. You test the solution before verifying your customers’ jobs, pains, and gains
If your test indicates that your solution doesn’t solve a problem, you won’t know if the solution is wrong or if the jobs, pains, and gains you’re trying to address doesn’t exist. You have to understand what jobs, pains, and gains influences customer behaviors before trying to verify if a solution address their priorities. Our Value Proposition Canvas was designed to avoid this very trap. The tool encourages innovators and entrepreneurs to test the circle before the square.
2. Your testing environment differs from real life situations
Your test is a lens to understand the real world and verify if your idea can work within it. If you test your assumptions under conditions that don’t match the real world, your results will be incorrect. For example: you’re testing customer interest for a diet right after Thanksgiving. The high interest you receive validates that losing weight is a crucial job for your customer, but only based on the data you got at that point in time. This job might not be as important for your customer during the rest of the year when customers’ are outside of special holiday seasons.
3. You value opinions over evidence
There is a difference between surveys (indicate what the customer wants to tell you) and calls-to-actions (indicate how people actually behave). Interviews and surveying are great ways to get rich insights when you get started, but can be extremely unreliable because customers often don’t act like they say they do. To validate critical assumptions, you need to test if people behave the way they say they would with a call-to-action (CTA). You can learn and discover unexpected opportunities or potential risks by getting customers to interact with a minimum viable product (MVP) like a landing page or online ad rather than hearing them comment on your idea.
4. Your Call-To-Action (CTA) is weak and fails to reveal a real interest or preference
Time, money, and reputation are three things that people aren’t willing to sacrifice easily. Your call-to-action truly shows a strong interest or preference only if customers are willing to spend their money and time, or risk their reputation by participating in your CTA.
5. You chose the wrong testing technique
There is a disconnect between what you need to learn and the test you chose to uncover those insights. For example, Dropbox (file synchronization company) initially tried to test interest in its app through Google ads to see if people would search for their type of solution. But because they were in a new market without a lot of users and existing competitors, people weren't in a mind frame to search for Dropbox online. The business environment clearly showed that customers had jobs, pains, and gains in this area, but the testing technique that Dropbox used failed to verify the existence of those pains.
6. You chose the wrong ‘success metrics’ to validate what is needed for your business model to work
When you verify your assumption via an experiment, you set up your test with a set of 'success metrics' to determine if a test is validated or invalidated. How do you know how much is enough to consider a test as validated? Is there a disconnect between what you need to learn and what you consider as ‘validation’? What do you consider as successful market share? What revenues do you need to sustain your business? You can research benchmarks to get a rough idea, but it’s also important to go back to your business model and define success metrics based on what is needed for your business to work.
7. You didn’t test business killers first and instead focused on hypotheses with the least impact
You will take more time to learn if your idea is promising if you start by testing the hypotheses that have less impact on the viability of your business. You're much better off by first verifying the crucial hypotheses that need to be true for your idea to work, especially assumptions that could undermine your business if you’re wrong.
8. You forgot to invalidate your hypothesis and remove your own bias
You choose a hypothesis that can’t be falsified or proven wrong. It’s tempting to conclude that a test is validated when data is ‘promising.' But you might also be hallucinating. Seek the invalidation of your idea by verifying the opposite of your hypothesis to avoid biased conclusions.
9. You test too many things at once, making it hard to understand the outcomes
If you test too many variables at once, you risk misinterpreting data or having to run additional tests to make a decision. For example: your flyer displays an image of your product, as well as its price and a phone number to call if people are interested in buying it. But people don’t call. How do you know if they are not interested, or if they are shying away from an expensive product? The data isn’t actionable, and you’re not learning from this test.
10. You don’t adapt the sample size to your testing context
The larger the sample, the more accurate the data. But the larger the sample, the longer the learning cycle. At the early stages, get insights as quickly as possible by testing with a smaller sample. Find patterns and resonance, and then test your idea with an MVP on a larger sample. When testing with a smaller sample, always keep in mind that the data could be flawed.
11. You give too much or too little time to your test
You don’t give enough time to your test to produce results - or you wait for too long. Is the additional time spent on this test worth the amount of learning?
12. Your commitment to the original idea prevents you from discovering superior alternatives
You disregard data that could indicate there’s a better opportunity than the idea you’re testing because you’re in love with your original idea. Stay as detached and as adaptable as you can because a superior alternative might exist.
13. You’ve poorly executed your tests and the data is flawed
Your data could be flawed if the test is poorly executed. An ad of poor quality will not get clicked on even if customers are interested in that kind product.
Innovators and entrepreneurs that design their tests with these “traps” in mind are likely to see a clear and accurate picture of their environment. Based on this data, they are able to figure out whether their business could potentially succeed. But using tests to answer questions will only take you so far. Instead, the most successful entrepreneurs use data to ask the right questions and continue to explore where the best opportunities lie.